The rapid advancement of artificial intelligence has ushered in a new era of transformative potential across industries, with AI-powered healthcare leading the way. A recent study published on arXiv introduces a groundbreaking development: the Health LLM architecture. Designed to tackle the complexity of accurate medical diagnoses, Health LLM outperforms existing models like GPT-3.5 Turbo and GPT-4, setting a new benchmark with an 83.3% diagnostic accuracy rate.
In this article, we explore the study, its implications, and the exciting trajectory of AI-powered healthcare diagnostics.
The Challenge of Medical Diagnosis
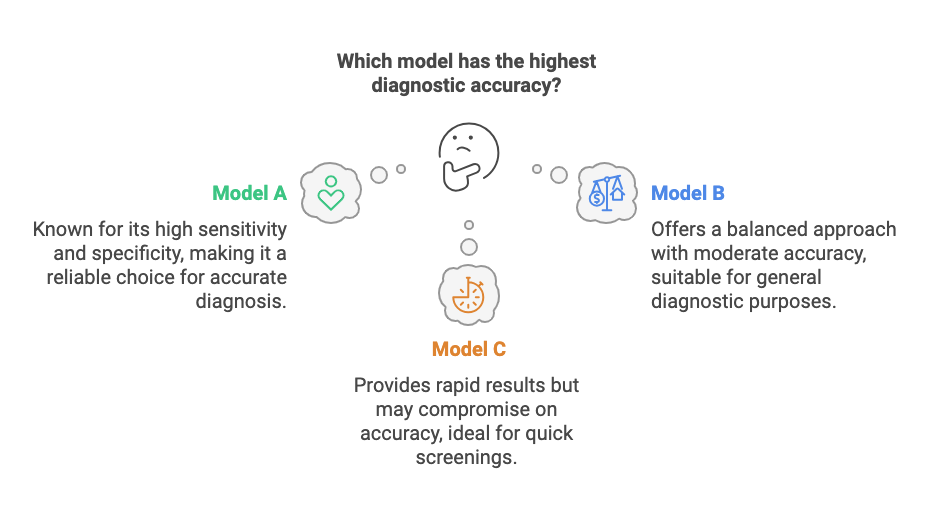
Medical diagnosis is a multifaceted and nuanced process. Accurate diagnosis demands a holistic analysis of symptoms, patient history, contraindications, and often subtle nuances discernible only to experienced clinicians. Healthcare professionals dedicate years to mastering these intricacies through extensive training and memorization of vast datasets and diagnostic protocols.
This complexity underscores the transformative potential of AI models like Health LLM, which integrate human-like reasoning with advanced computational power to address these challenges.
The Health LLM Architecture: A Closer Look
Health LLM employs a sophisticated architecture that combines multiple state-of-the-art techniques to simulate the diagnostic processes of medical professionals. Key components include:
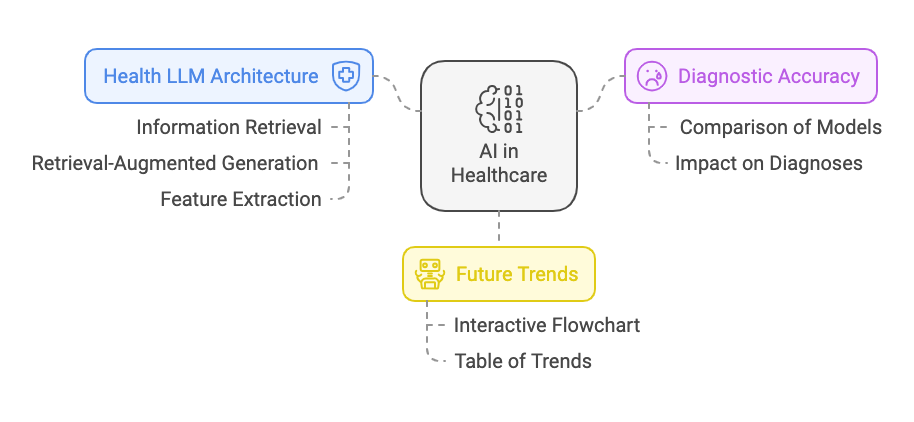
1. Information Retrieval
The system connects to extensive medical databases, including peer-reviewed literature, clinical guidelines, and patient registries, to retrieve relevant knowledge.
2. Retrieval-Augmented Generation (RAG)
RAG enhances diagnostic accuracy by integrating external knowledge with in-context learning from the patient’s data.
3. Feature Extraction
The model extracts actionable features from patient data, such as symptoms, medical history, family history, medications, allergies, and lab results.
4. Question Answering
By employing internal mechanisms, Health LLM simulates counterfactual reasoning, exploring “what-if” scenarios to refine diagnostic predictions.
5. Machine Learning Integration
The architecture incorporates advanced ML models like XGBoost alongside sequence-to-sequence transformers to analyze structured and unstructured data effectively.
Results That Redefine Accuracy
Health LLM demonstrated remarkable accuracy when compared to leading AI models:
- GPT-3.5 Turbo: Achieved 33% diagnostic accuracy.
- GPT-4 with Few-Shot Learning: Improved to 66%.
- Health LLM: Surpassed both with 83.3% accuracy.
Notably, the inclusion of sophisticated information retrieval techniques improved diagnostic accuracy by 5%, highlighting the critical role of integrating external knowledge.
Why This Matters: Implications for Healthcare
The implications of Health LLM’s success extend far beyond academic validation. It signals transformative potential in key areas:
1. Accelerated Diagnoses
AI can analyze complex patient data in seconds, enabling quicker diagnostic timelines—critical for time-sensitive conditions.
2. Enhanced Diagnostic Accuracy
While not yet sufficient for standalone deployment, Health LLM’s trajectory suggests near-perfect accuracy could be achievable within a decade.
3. Addressing Healthcare Inequities
With widespread adoption, Health LLM can democratize access to quality diagnostics, especially in underserved regions.
4. Personalized Medicine
Incorporating patient history, genetic data, and lifestyle factors enables highly tailored diagnostic and treatment plans.
Challenges and Opportunities
Limitations of the Study
While promising, the study faces certain limitations:
- Narrow Dataset: The model was tested on only 61 diseases, whereas the ICD-11 catalog contains over 17,000 potential diagnoses. Expanding datasets is essential.
- Exclusion of Comprehensive Data: The current architecture does not yet incorporate patient charts, genetic profiles, or imaging data. Integrating these would significantly enhance accuracy.
- Ethical Concerns: Bias, privacy issues, and algorithmic fairness need to be addressed for real-world applicability.
Future Directions
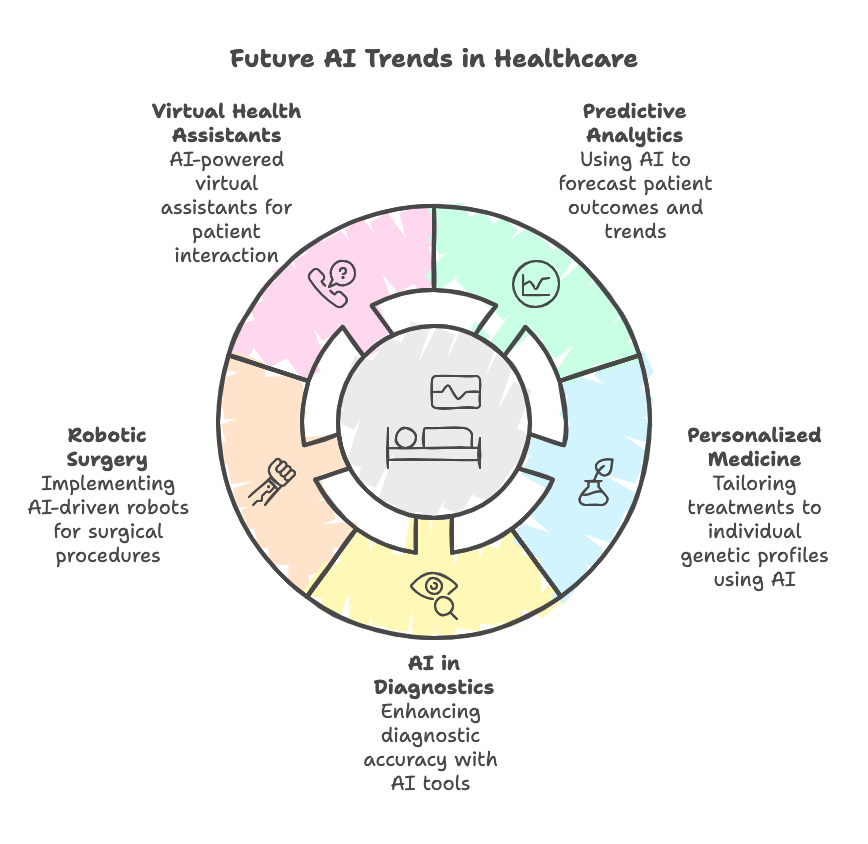
- Explainable AI (XAI): Adding transparency to the decision-making process would help clinicians understand and trust the model’s recommendations.
- Multimodal Data Integration: Incorporating diverse data types such as medical imaging, audio (heart and lung sounds), and real-time vitals would improve diagnostic depth.
- Human-in-the-Loop Systems: Emphasizing collaborative systems where AI supports, rather than replaces, human judgment ensures safer and more reliable outcomes.
AI in Healthcare: The Bigger Picture
Health LLM is one of many innovations transforming healthcare. Here’s a look at other impactful AI applications:
- Drug Discovery: Accelerating the identification and development of new therapies.
- Predictive Analytics: Anticipating patient outcomes and optimizing hospital resource allocation.
- Robotic Surgery: Enhancing precision and minimizing invasiveness.
- Telemedicine: Enabling remote consultations and diagnostics.
- Personalized Treatment Plans: Customizing therapies to individual patient profiles.
How to Stay Ahead in AI Healthcare Careers
The rise of AI in healthcare is creating opportunities across various roles. To thrive in this evolving field:
- Develop AI Expertise: Master platforms like TensorFlow and PyTorch, and explore healthcare-focused tools.
- Understand Healthcare Systems: Learn about EHRs, medical coding, and clinical workflows.
- Focus on Ethical AI: Prioritize principles like fairness, accountability, and patient privacy.
- Embrace Lifelong Learning: Continuously update your knowledge as AI evolves.
Conclusion
The Health LLM study represents a pivotal moment in AI-powered diagnostics, bridging the gap between human expertise and machine intelligence. As AI technology advances, the potential for faster, more accurate, and equitable healthcare solutions grows exponentially.
By embracing these innovations, addressing challenges, and fostering interdisciplinary collaboration, we stand on the brink of a healthcare revolution.
Read more
- About the Health LLM Study:
- Link: arXiv: The Health LLM Study (Link to the specific study or preprint if available)
- Placement: When discussing the accuracy results of Health LLM.
- Example: “A recent study published on arXiv highlights the breakthrough performance of Health LLM in medical diagnostics.”
- ICD-11 Classification:
- Link: World Health Organization: ICD-11
- Placement: When discussing the limitations due to the limited number of diseases tested.
- Example: “Considering the ICD-11’s classification of over 17,000 diseases, further expansion of Health LLM’s training data is essential.”
- Ethical AI in Healthcare:
- Link: AI Ethics Guidelines by the WHO
- Placement: In the ethical considerations section.
- Example: “Adopting ethical principles, as outlined by the World Health Organization’s AI Ethics Guidelines, is essential to ensure trust in AI systems.”
- AI in Healthcare Trends:
- Link: McKinsey Report on AI in Healthcare
- Placement: When discussing the broader impact of AI on healthcare.
- Example: “According to a McKinsey report, AI is poised to transform diagnostics, treatment personalization, and operational efficiency.”
- AI-Powered Drug Discovery:
- Link: Nature Article on AI Drug Discovery
- Placement: In the AI in Healthcare section, under drug discovery.
- Example: “AI-driven drug discovery has accelerated timelines, as demonstrated in this Nature article.”
- AI Learning Resources:
- Link: Coursera AI Courses (or another relevant resource)
- Placement: In the “How to Stay Ahead in AI Healthcare Careers” section.
- Example: “For those looking to upskill, platforms like Coursera offer comprehensive courses in AI and machine learning.”
Explore More
- AI Services: Explore our AI services for more details.
- Digital Product Development: Discover our digital product development expertise.
- Design Innovation: Learn about our design innovation approach.
Leave a Reply